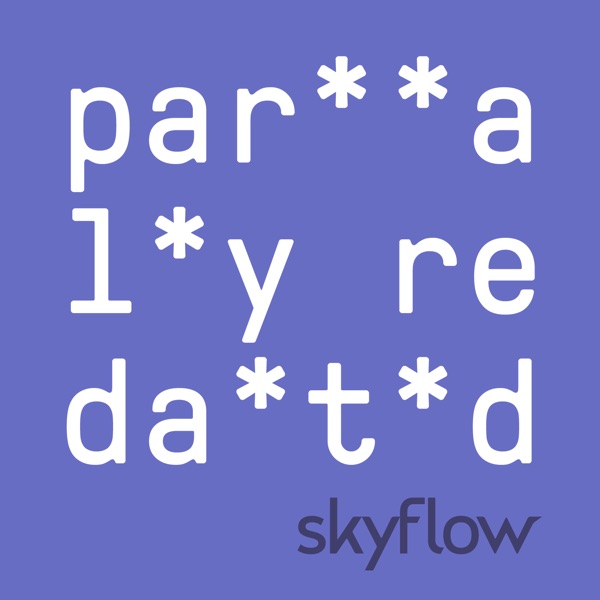
Partially Redacted: Data, AI, Security, and Privacy
Skyflow
Podcast
Episodes
Listen, download, subscribe
Prompt Injection Attacks with SVAM's Devansh
• 47 minIn this episode, we dive deep into the world of prompt injection attacks in Large Language Models (LLMs) with the Devansh, AI Solutions Lead at SVAM. We discuss the attacks, existing vulnerabilities, real-world examples, and the strategies attackers use. Our conversation sheds light on the thought process behind these attacks, their potential consequences, and methods to mitigate them. Here's what we covered: Understanding Prompt Injection Attacks: A primer on what these attacks are and why they pose a significant threat to the integrity of LLMs. Vulnerability of LLMs: Insights into the inherent characteristics of LLMs that make them susceptible to prompt injection attacks. Real-World Examples: Discussing actual cases of prompt injection attacks, including a notable incident involving DeepMind researchers and ChatGPT, highlighting the extraction of training data through a clever trick. Attack Strategies: An exploration of common tactics used in prompt injection attacks, such as leaking system prompts, subverting the app's initial purpose, and leaking sensitive data. Behind the Attacks: Delving into the minds of attackers, we discuss whether these attacks stem from a trial-and-error approach or a more systematic thought process, alongside the objectives driving these attacks. Consequences of Successful Attacks: A discussion on the far-reaching implications of successful prompt injection attacks on the security and reliability of LLMs. Aligned Models and Memorization: Clarification of what aligned models are, their purpose, why memorization in LLMs is measured, and its implications. Challenges of Implementing Defense Mechanisms: A realistic look at the obstacles in fortifying LLMs against attacks without compromising their functionality or accessibility. Security in Layers: Drawing parallels between traditional security measures in non-LLM applications and the potential for layered security in LLMs. Advice for Developers: Practical tips for developers working on LLM-based applications to protect against prompt injection attacks. Links: Devansh on LinkedIn AI Made Simple
Partially Redacted: Data, AI, Security, and Privacy RSS Feed